- 1Burden of Disease Research Unit, South African Medical Research Council, Cape Town, South Africa
- 2School of Public Health, University of the Western Cape, Cape Town, South Africa
- 3Division of Health Systems and Public Health, Department of Global Health, University of Stellenbosch, Stellenbosch, South Africa
Objectives: The present study examined the prevalence and patterns of non-communicable disease multimorbidity by wealth quintile among adults in South Africa.
Methods: The South African National Income Dynamics Study Wave 5 was conducted in 2017 to examine the livelihoods of individuals and households. We analysed data in people aged 15 years and older (N = 27,042), including self-reported diagnosis of diabetes, stroke, heart disease and anthropometric measurements. Logistic regression and latent class analysis were used to analyse factors associated with multimorbidity and common disease patterns.
Results: Multimorbidity was present in 2.7% of participants. Multimorbidity was associated with increasing age, belonging to the wealthiest quintile group, increasing body mass index and being a current smoker. Having secondary education was protective against multimorbidity. Three disease classes of multimorbidity were identified: Diabetes and Hypertension; Heart Disease and Hypertension; and Stroke and Hypertension.
Conclusion: Urgent reforms are required to improve health systems responsiveness to mitigate inequity in multimorbidity patterns in the adult population of South Africa as a result of income inequality.
Introduction
South Africa is an upper-middle-income country [1], with one of the highest levels of inequality in the world [2]. The country has a quadruple burden of disease; with mortality trends illustrating that 43% of deaths were due to non-communicable diseases (NCDs), 34% to HIV/AIDS and TB, 14% to other communicable diseases (and perinatal conditions, maternal causes and nutritional deficiencies) and 10% to injuries [3]. It is currently observed that NCDs disproportionately affect people in low and middle-income countries (LMICs), where 85% of premature deaths due to NCDs occur [4].
The observed large increases in NCD burdens in sub-Saharan Africa are driven by the increase in cardiovascular risk factors (i.e., unhealthy diets, physical inactivity, obesity and air pollution) [5]. Multimorbidity is the co-existence of multiple health conditions in an individual [6]. It is reported that one in three people are living with multimorbidity globally [7]. Multimorbidity is associated with increases in healthcare costs and utilization, medication use, hospital admissions, and out of pocket healthcare costs [8]. Individuals with multimorbidity have higher mortality risk [9], a poorer quality of life [10], and complicated medication adherence requirements [11]. The prevalence of NCD multimorbidity in LMICs is already estimated to be as high as 36%. A scoping review highlighted the urgent need for a better understanding of the epidemiology of multimorbidity in LMICs to inform interventions to improve the outcomes of patients with living with multiple diseases [12].
The literature on multimorbidity and socioeconomic status remains divided; possibly due to the way in which multimorbidity is operationalised, as well as varying contexts (e.g., high versus low income countries), and the different ways in which socioeconomic status, wealth and deprivation are measured. A systematic review (focused mainly on high income countries) found increasing levels of deprivation associated with increases in multimorbidity [13]. In cross-sectional studies in high income countries (i.e., South Korea [14] United States, Canada [15], England [15, 16] and Ireland [15]) and China [17] the prevalence of multimorbidity was highest in those with a low socioeconomic status. In contrast, there are reports that multimorbidity could be more prevalent among wealthier people in LMICs [18]. This paper thus reports on the prevalence and patterns of NCD multimorbidity by wealth quintile in a household panel survey of adults in South Africa.
Methods
Description of the National Income Dynamics Study Survey Sample and Data Collection
The South African National Income Dynamics Study (NIDS) is the first national household panel study in South Africa that provides information on wealth (livelihoods, how households cope with shocks, poverty, and social capital), sociodemographic (household composition, fertility and mortality, migration, economic activity and education), and health and well-being characteristics [19]. We used data from NIDS Wave 5 conducted in 2017. Health data on diabetes, stroke and heart disease were collected through self-report, and blood pressure and anthropometry measurements were taken at survey administration.
The baseline data collection of the NIDS survey was conducted in 2008 [20], when a two-stage cluster sample design was used to randomly select about 7,300 households across 400 primary sampling units, stratified by district council (a second level administrative division of South Africa’s territory into 52 areas). Data were collected on all members of the selected households, resulting in a total sample size of approximately 28,000 individuals [21]. In the following waves of data collection the same individuals (continuing sample members) were recontacted and interviewed. In addition, all adults belonging to the same household of the continuing sample members at the moment of the interview (temporary sample members) were also interviewed. A top-up sample was recruited in during Wave 5 to compensate for sample attrition and improve representativity of the national population.
This study uses data from Wave 5 of the survey, where 39,400 individuals in 10,800 households were interviewed between February and December 2017 [22]. Additional methodological details from the NIDS Wave 5 survey are available in the Panel User Manual [23].
Ethics approval for data collection for NIDS Wave 1 to 5 was granted by the University of Cape Town’s (UCT) Commerce Faculty Ethics in Research Committee and Faculty of Health Sciences Human Research Ethics [24]. Informed consent in the respondent’s preferred language was obtained for all data collection in the survey. Permission and access to the edited and anonymised dataset (available for public distribution) was obtained from the research data service, DataFirst [24]. The current analysis was approved as part of the lead author’s doctoral studies and received additional ethics clearance by the Biomedical Research Ethics Committee of the University of the Western Cape (BM20/5/8).
Measures
Outcome Variable: Multimorbidity
This study employed a count method for assigning multimorbidity, by counting the number of co-existing disease conditions using a pre-defined list [25]. We created the list of included disease conditions based on recommendations by Holzer et al. [26]. The disease conditions included were based on a predefined list of disease conditions that are frequently included in multimorbidity assessments and disease conditions that are relevant to the South African disease burden. We included self-reported and measured disease conditions. For self-reported diseases, those that could be deemed as “current” were included. For the current analysis study, we included diabetes, heart disease, stroke and hypertension.
For each participant, an index variable was created which added up the number of disease conditions present for each person. If there was missing information for a disease condition, the observation was assumed to have “no disease present.” The Multimorbidity Index was then created by classifying the index variable into those with no disease conditions or one disease condition (i.e., “no multimorbidity”) or those with two or more disease conditions (i.e., “multimorbidity present”).
Self-Reported Disease Conditions
Participants were asked if they were ever told by a doctor, nurse or health care professional that they had the disease condition (i.e., diabetes, heart disease and stroke) (Supplementary Table S1). The responses were coded in a binary manner (e.g., disease absent or present).
Blood Pressure
For blood pressure measurements, duplicate measurements in the left arm after the participant was seated for at least 5 min [21]. Automated oscillometric devices with standard multi-size cuffs were used to take blood pressure measurements [21]. The average of the replicated readings was considered as the subject’s blood pressure. Replicated measurements of systolic and diastolic blood pressure were assessed for the presence of implausible values (systolic BP < 70 mmHg or >270 mmHg, diastolic BP < 30 mmHg or >150 mmHg), which were set to missing. Hypertension was grouped into “no hypertension present” (systolic BP < 120–139 mmHg & diastolic BP < 80–89 mmHg) or “hypertension present” (systolic BP 140 to ≥160 mmHg & diastolic BP 90 mmHg to ≥100 mmHg) [27]. People on hypertensive medication were included in those that had hypertension.
Sociodemographic and Lifestyle Risk Factors
The following variables were investigated as predictor variables of multimorbidity based on the literature [28]: age, sex, locality, educational attainment, employment status, income, asset index, access to medical aid, smoking status and body mass index (BMI).
Employment status was derived using the script available from the NIDS study [23] and based on the International Labour Organization’s definitions of employed, unemployed (strict definition), unemployed (broad definition) and not economically active [23]. Individual income was split into three categories, with the first representing no income and the third representing the highest income.
An asset-based wealth index, based on the 2016 South African Demographic and Health Survey [29, 30], was constructed. The index was created using principal component analyses of questions on:
(a) access to basic services (e.g., household main source of water, type of toilet facility, main source of energy for heating and cooking, and refuse collection),
(b) housing (e.g., number of people living in the dwelling per room, dwelling type, home ownership, material of roof, walls and floors) and
(c) ownership of durable assets (e.g., household has electricity, radio, television, phone, computer, fridge, microwave, gas/electric stove, washing machine, cellphone, bicycle, motorbike, motor vehicle, animal cart and boat).
Each variable was coded as binary (i.e., present or absent), except for the number of people in household per room. Scores were predicted for each household and these scores were then divided into five wealth quintiles—with the 1st representing the lowest quintile (i.e., least wealthy) and the 5th representing the highest quintile (i.e., most wealthy). Each individual in the household was assigned the same quintile.
Anthropometry
The NIDS Wave 5 assessed participants based on height and weight measurements taken using a digital scale and stadiometer. The data cleaning followed the procedure used for the BMI risk factor in the second South African Comparative Risk Assessment Study [31]. Implausible values were considered as missing. BMI was calculated using the BMI STATA package and was categorized as follows: underweight (15.0 to <18.5 kg/m2), normal weight (18.5 to <25.0 kg/m2), overweight (25.0 to <30.0 kg/m2), obesity grade 1 (30.0 to <35.0 kg/m2), obesity grade 2 (35.0 to <40.0 kg/m2), obesity grade 3 (40.0 to <60.0 kg/m2) [31].
Statistical Analysis
Statistical analyses were conducted using STATA 15.0 (Stata Corporation, College Station, TX, United States) software. To account for the complex survey design of the NIDS, including clustering, stratification and unequal selection probability, the STATA survey set (“svy”) of commands were used. Sampling weights calibrated to the represent the South African demographics were used as provided in the original dataset [32]. Weighted data exploration was conducted. Chi-square tests were used to explore bivariate associations between the wealth index quintile and sex, locality, province, educational attainment, employment status, BMI categories and smoking status. Kruskal-Wallis tests were used to test for differences in age and income between the different quintiles in the wealth index. Similarly, Chi-square tests were used to assess single disease conditions and the number of diseases in an individual by wealth index quintile. Multimorbidity status was also described using histograms and box plots against age.
Multivariate logistic regression was employed to assess the relationship between multimorbidity and potential predictors (i.e., age, sex, location, educational attainment, employment, income, wealth index, smoking status and BMI). For the regression, age was categorised according to the United Nations guidelines for age classifications (i.e., 15–24 years, 25–44 years, 45–64 years and 65+ years) [33]. The crude odds ratios were estimated for each predictor variable. The final model included all variables. Model-checking was performed using various statistical tests. The link test [34] was used to determine if there were specification errors. Interaction terms were explored. Pearson residuals, deviance residuals and Pregibon leverage were used to assess influential observations [35]. These tests were done on the unweighted model as they cannot be used on survey weighted data. Crude and adjusted odds ratios were reported with 95% confidence intervals (Cis); p-values of less than 0.05 were considered statistically significant.
A latent class analysis (LCA) was performed to explore disease clustering with the four selected disease conditions (i.e., diabetes, stroke, heart disease, hypertension). LCA is a statistical method used to identify sub-groups or classes within populations [36]. The analysis was run using the LCA Stata Plugin as the programme accounts for complex survey design [37, 38]. We conducted the LCA as recommended by Weller et al. [36] For example, to identify latent classes, a one-class model was estimated and then additional classes were added to compare the relative fit of models [36]. The relative fit of models were compared using a series of information indices, namely, the Bayesian information criterion (BIC) [39], the adjusted BIC (aBIC) [40], and the Akaike Information Criterion (AIC) [41]. Lower values of these information indices indicated a better fit [42]. After selecting the model with the best fit, individuals were assigned to the class with the highest posterior probability.
Results
Sample Description
The sample consisted of 27,042 participants, with more females (56.9%, n = 15,362) than males (Table 1). The median age of the sample was 33 years (IQR: 23–51). In terms of population group, most of the sample were Black African (77.9%), followed by Coloured (13.5%), White (6.5%) and Asian (2.1%). Missing data is reported in Supplementary Table S2.
Just more than half of the sample lived in an urban location (55.7%). Place of residence varied significantly by wealth quintile, with a high proportion of wealth Quintile 1 living in rural areas (83.9%) and a large proportion of Quintile 5 living in urban areas (89.5%).
Approximately 63% of participants completed secondary education, and tertiary educational attainment was highest in wealth Quintile 5 group. Only a third of the sample was employed and income levels varied by wealth quintiles. Income was heavily skewed to the left with median income being R0 in all quintiles (overall interquartile range: R0–R2000). Approximately 11% of the sample had access to private medical aid, and significantly higher in wealth Quintile 5.
About 42% of the sample had a normal BMI, with females significantly more likely to fall outside the normal BMI range (p < 0.001) (Supplementary Table S3). Levels of obesity was highest in wealth Quintile 5. Current smoking status varied by quintiles and peaked in the wealth Quintile 4 group.
Disease Prevalence in the Population
When taking population weighting into account, hypertension was the most prevalent disease condition in the population (27.8%, 95% CI: 26.7–29.0) (Figure 1). This was followed by diabetes (2.9%, 95% CI: 2.6–3.3), heart disease (1.6%, 95% CI: 1.3–1.8) and stroke (0.8%, 95% CI: 0.6–1.0). The prevalence of hypertension and diabetes rose with increasing wealth quintiles (Supplementary Table S4). The prevalence of heart disease was similar in wealth Quintiles 1–3 but increased in Quintile 4 and 5. The prevalence of stroke did not appear influenced by wealth quintile.
The prevalence of hypertension was similar among males and females (Supplementary Table S4). Hypertension prevalence increased with increasing age and peaked in the 70+ year age group at 70.6% in males and 73.6% in females (Supplementary Figure S1). Diabetes, heart disease and stroke started increasing in the 40–49-year age group and peaked in the 70+ year age group.
The majority of the population had none of the included diseases (74.5%, 95% CI: 73.5–75.4) (Table 2). A further 22.8% (95% CI: 21.8–23.8) had one disease condition. Multimorbidity was present in 2.7% (95% CI: 2.4–3.1) of the population, with it being more prevalent among wealth Quintile 5 compared to the other quintiles. Multimorbidity was also more prevalent among females compared to males (Supplementary Table S6). The prevalence of multimorbidity was low in younger age groups and peaked at 13.2% among the 70+ age group (Supplementary Figure S1).

TABLE 2. Number of disease conditions in individuals by wealth index (South Africa, 2017, weighted).
Factors Associated With Multimorbidity
Multimorbidity was strongly and significantly associated with age, with the odds of having multimorbidity increasing rapidly among older age groups (Table 3). In the crude analysis, females had higher odds of multimorbidity compared to males, but this did not remain significant in the adjusted model. Locality was not associated with multimorbidity.
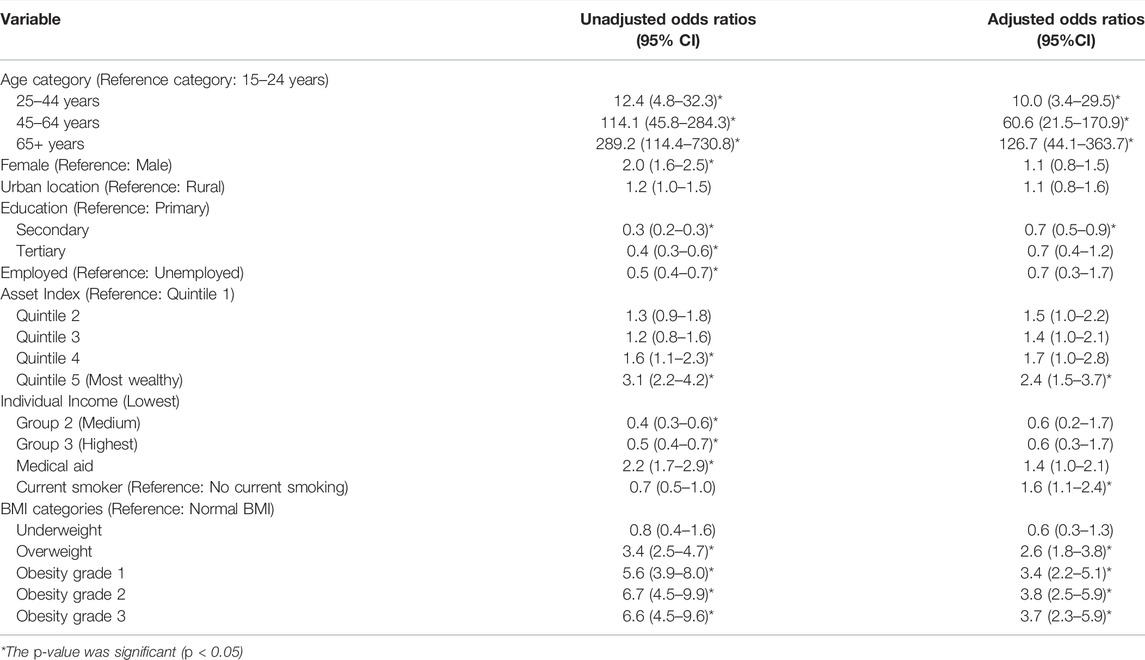
TABLE 3. Factors associated with multimorbidity (crude and adjusted Odds Ratios, South Africa, 2017).
Having secondary education was associated with reduced odds of multimorbidity, compared to those with only primary school education (OR: 0.7, 95% CI: 0.5–0.9). While a similar pattern was observed in those with tertiary education, it was not significant in the adjusted analysis—possibly due to a lack of statistical power. People in Quintile 5 (most wealthy) had 2.4 times the odds of having multimorbidity compared to those in Quintile 1 (least wealthy) (95% CI: 1.5–3.7). In the adjusted analysis, there was no significant association between multimorbidity and income, having medical aid or being employment.
When compared to those with normal BMIs, those that were overweight had more than double the odds of multimorbidity (OR: 2.6, 95% CI: 1.8–3.8), those with obesity grade 1 had three times the odds of multimorbidity (OR: 3.4, 95% CI: 2.2–5.1) and those with obesity grade 2 and 3 had almost four times the greater odds of multimorbidity (OR: 3.8, 95% CI: 2.5–5.9 and OR: 3.7, 95% CI: 2.3–5.9, respectively). Smokers were more likely to have multimorbidity than non-smokers (OR:1.6, 95% CI:1.1–2.4).
Multimorbid Population Analysis
Only the multimorbid sample was included in the subsequent analysis (n = 971). The unweighted mean age of people with multimorbidity was 60 years (58.9–61.5 years). Also, 67.7% were female. In terms of included disease conditions (weighted), almost all the multimorbid population had hypertension (98.9%, 95% CI: 97.6–99.5), followed by diabetes (68.2%, 95% CI: 63.0–72.9), heart disease (37.5%, 95% CI: 32.4–43.0) and stroke (16.1%, 95% CI: 13.2–19.4) (Supplementary Table S7). The disease prevalence was similar among the sexes, except for stroke which was more prevalent in males (20.1%, 95% CI: 14.4–27.4) compared to females (14.1%, 95% CI: 11.0–18.0).
Supplementary Table S8 shows a comparison of fit statistics for models with different numbers of classes, ranging from two to five classes. The BIC, adjusted-BIC and AIC were minimal for a three-class model. A four-class model produced a slightly lower AIC but since the AIC tends to prefer over-complicate models, the three-class model was chosen. Classes were named based on the diseases with the highest prevalence in that class. The model identified the following membership, latent classes, from largest to smallest: “Diabetes and Hypertension” (52.6%), “Heart disease and Hypertension” (32.1%), and “Stroke and Hypertension” (15.3%) (Figure 2). A high probability of hypertension was common among all the disease classes. Standard errors are available in Supplementary Table S9.
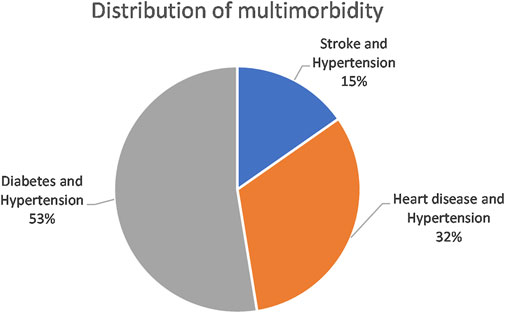
FIGURE 2. Distribution of latent classes in the multimorbid population (South Africa, 2017, weighted).
Table 4 shows the item response probabilities for each disease condition by latent class. The largest class (Diabetes and Hypertension) was characterised by 100% certainty of having both diabetes and hypertension. The second-largest class (Heart disease and Hypertension) was typified by a 99.9% probability of heart disease and a 98.4% probability of hypertension. The smallest class (Stroke and Hypertension) was characterised by very high probabilities of stroke (99.7%) and hypertension (95.6%).

TABLE 4. Disease probabilities within classes for the 3-class latent class analysis model (South Africa, 2017).
Discussion
Our results support the well-established notion of a positive association between increase in age and NCD multimorbidity; as shown in a recent systematic review of multimorbidity in 22 out of 25 studies in LMICs [43]. South Africa’s population is ageing—the proportion of elderly persons (60 years and older) increased from 7.6% in 2002 to 9.1% in 2020 [44]—and signals the need to anticipate the needs of an aged population. Further, the National Development Plan 2030 that aims to increase life expectancy of South Africans from 61 to 70 years, propagates for integrated health care delivery through the life course [45]. The life-course approach increases the effectiveness of interventions by targeting the needs of individuals at critical points in their lives [46]. In South Africa, screening older adults for multimorbidity will enable the identification of individuals needing treatment.
Our study found a positive association between multimorbidity and the highest wealth quintile. Studies on the African continent (e.g., Burkino Faso [47], Ghana [17], South Africa [48]) have shown that wealthiest groups had higher levels multimorbidity compared to the less wealthy groups. This contrasts with what has been noted in high income countries. In LMICs, this could be explained by wealthier people having access to high-calorie foods, tobacco, alcohol and other factors that can increase the risk of developing multiple conditions [18]. It is possible that residual confounding existed in our regression model and we were not able to fully separate the effects of wealth on education, occupation and BMI. Of course, the relationship with wealth may be mediated or confounded by education and occupation. Wealth and prosperity tends to be related to higher BMI in South Africa [49, 50], a factor also associated with multimorbidity. Obesity risk in African population groups may also be influenced by cultural norms that associate fatness with beauty [51].
Access to healthcare may also contribute to explain the association between multimorbidity and wealth. Since South Africa has both private and public health sector systems [52], wealthier people tend to have better access to healthcare in terms of private health insurance or medical aid. For example, many private medical aid schemes in the country offer—or even require mandatory—annual health screening benefits (blood pressure, BMI, glucose and cholesterol), which would likely increase awareness of these health conditions among those on higher wealth quintiles.
The common disease classes identified among the multimorbid population were “Diabetes and Hypertension,” “Heart disease and Hypertension” and “Stroke and Hypertension.” The largest disease class in our study was “Diabetes and Hypertension.” This combination of diseases has been previously identified in the literature both regionally [53–56] and internationally [57]. A multi-country study based in sub-Saharan Africa found that hypertension was the most common co-morbidity in a cohort of diabetes patients and was present in approximately 71% of patients with diabetes [58]. An analysis of a South African national HIV survey also identified “Diabetes and Hypertension” and “Heart disease and Hypertension” as disease classes in the multimorbid population [59]. Hypertension is a condition in which blood vessels have persistently raised pressure and if left untreated, can cause chest pain (angina), heart attacks, heart failure, and an irregular heartbeat, which can lead to a sudden death [60]. It can also cause strokes by blocking or bursting arteries that supply blood or oxygen to the brain [60]. Hypertension has many short and long term consequences [61]—such as the disease conditions identified as co- or multi-morbid in this study (diabetes, stroke, heart disease or heart failure). The number of people with hypertension is increasing and detection but treatment rates remain strikingly low (control rates are below 13% in Sub-Saharan Africa) [62]. While prevention and screening are important, of equal importance is the need to effectively manage patients. To reduce fragmentation of care and meet the needs of people with multimorbidity, several European countries have introduced disease management programmes focused on integrated care [63]. The World Health Organization has also suggested that integrated care is beneficial for older people [64]. Given the growing burden of NCDs in many LMICs, integrated care aimed at reducing and managing the burden of NCDs need to be investigated.
Limitations
This study was a secondary analysis of survey data and was limited to the data reported in the survey. Since the NIDS 2017 survey collected information on few disease conditions, this analysis was also limited in the number of disease conditions included. We used a combination of measured and self-reported health data. The self-reported disease condition data was most likely underestimated which would, in turn, lead to an underestimation in the prevalence of multimorbidity. We also included a few disease conditions which may also lead to an underestimation in the assessed prevalence of multimorbidity. In addition, we assumed that missing data for a disease condition meant no disease was present which may have led to a lower estimation of prevalence for disease conditions. The prevalence of multimorbidity in this study was lower compared to other local studies [53]. However, some multimorbidity prevalence studies that included younger people in South Africa also found lower prevalences i.e. between 6 and 13% [59, 65, 66]. The prevalence in this study was very similar to the prevalence estimates observed in another study of the 2008 NIDS Wave 1 (4.0%) [48] and the 2010 NIDS Wave 3 (2.8%) [67]. This could be due to the NIDS being a panel survey that goes back to interview a similar panel of participants. It is also most likely due to the underestimated prevalence of self-reported single diseases found in all three studies based on the NIDS surveys. While each study included variations of disease conditions, all three studies included hypertension and diabetes.
The estimated hypertension prevalence in this analysis appears to be plausible. For example, in this study, approximately 28% of the population 15 years and older was found to have hypertension (27.3% in males and 28.4% in females). In comparison, a meta-regression of hypertension estimates for the population 25 years and older was found to be 38.9% for males and 40% for females [68]. However, the diabetes prevalence in this study was 2.9% whereas other national surveys of people aged 15 years and older (using biomarkers) have placed the diabetes prevalence closer to 14.7% in 2012 [69] and 14.9% in 2016 [29]. A meta-regression of diabetes prevalence in people 25 years and older determined the prevalence to be 12.8% [70]. This indicates that the diabetes prevalence was most likely underestimated in the 2017 NIDS Wave 5, probably because it was self-reported. In a comparison of self-reported diabetes prevalence and prevalence based on HbA1c in another survey, the author concluded that 61% of cases of diabetes were likely to be undiagnosed [71]. As “Diabetes and Hypertension” make up the largest disease class in this study, any underestimation in the prevalence of these two diseases would have impacted the estimation of multimorbidity prevalence. While multimorbidity prevalence may be underestimated in our study, we still had important findings on the factors associated with multimorbidity and disease patterns.
Conclusion
This study builds upon previous studies that examined multimorbidity in earlier waves of the NIDS dataset. As in the previous studies, the multimorbidity prevalence remained low but this is most likely due to the under-reporting of disease conditions. There was still a substantial amount of morbidity in the population, especially due to hypertension which reached extremely high levels among older people. Our study highlighted that age, belonging to the highest wealth quintile, current smoking, being overweight or obese increased the odds of multimorbidity; whereas having secondary education was protective of multimorbidity. Slightly more than half of the participants with multimorbidity were estimated to belong to the “Diabetes and Hypertension” class. Integrated models of care are needed to prevent, manage and optimise the treatment of NCD multimorbidity.
Data Availability Statement
The NIDS 2017 data is available on the DataFirst website (https://www.datafirst.uct.ac.za/dataportal/index.php/catalog/712).
Ethics Statement
This study was a secondary data analysis of an anonymized dataset. The anonymized dataset was obtained from DataFirst with the necessary permissions. Ethics clearance to conduct this study was also granted by the Biomedical Research Ethics Committee of the University of the Western Cape (BM20/5/8). Written informed consent to participate in this study was provided by the participants.
Author Contributions
All authors conceptualised the manuscript. Data analysis was conducted by RR and AC. RR drafted the first version of the manuscript. All authors contributed to revising the manuscript and approved the final version.
Funding
The work reported herein was made possible through funding by the Burden of Disease Research Unit at the South African Medical Research Council. RR conducted this research under the South African Medical Research Council through its Division of Research Capacity Development under the Internship Scholarship Programme from funding received from the South African National Treasury.
Author Disclaimer
The content hereof is the sole responsibility of the authors and does not necessarily represent the official views of the South African Medical Research Council or the funders.
Conflict of Interest
The authors declare that the research was conducted in the absence of any commercial or financial relationships that could be construed as a potential conflict of interest.
Acknowledgments
We would like to acknowledge University of Cape Town’s Southern Africa Labour and Development Research Unit and DataFirst for access to the dataset. We would also like to thank Dr. Oluwatoyin Awotiwon and Prof. Ali Dhansay for their assistance with the inclusion of disease conditions.
Supplementary Material
The Supplementary Material for this article can be found online at: https://www.ssph-journal.org/articles/10.3389/ijph.2022.1605072/full#supplementary-material
References
1.The World Bank. Data: South Africa (2020). Available from: http://data.worldbank.org/country/south-africa (accessed May 24, 2022).
2.The World Bank. Gini index (World Bank Estimate) - South Africa (2022). Available from: https://data.worldbank.org/indicator/SI.POV.GINI?locations=ZA&most_recent_value_desc=true (accessed March 6, 2022).
3. Pillay-van Wyk, V, Msemburi, W, Laubscher, R, Dorrington, RE, Groenewald, P, Glass, T, et al. Mortality Trends and Differentials in South Africa from 1997 to 2012: Second National Burden of Disease Study. Lancet Glob Health (2016) 4(9):e642–53. doi:10.1016/S2214-109X(16)30113-9
4.World Health Organization. Noncommunicable Diseases (2021). Available from: https://www.who.int/news-room/fact-sheets/detail/noncommunicable-diseases (accessed March 6, 2022).
5. Bigna, JJ, and Noubiap, JJ. The Rising Burden of Non-communicable Diseases in Sub-saharan Africa. Lancet Glob Health (2019) 7(10):e1295–6. doi:10.1016/S2214-109X(19)30370-5
6. Almirall, J, and Fortin, M. The Coexistence of Terms to Describe the Presence of Multiple Concurrent Diseases. J Comorb (2013) 3(1):4–9. doi:10.15256/joc.2013.3.22
7. Nguyen, H, Manolova, G, Daskalopoulou, C, Vitoratou, S, Prince, M, and Prina, AM. Prevalence of Multimorbidity in Community Settings: A Systematic Review and Meta-Analysis of Observational Studies. J Comorb (2019) 9:2235042X19870934–15. doi:10.1177/2235042X19870934
8. Hajat, C, and Stein, E. The Global Burden of Multiple Chronic Conditions: a Narrative Review. Prev Med Rep (2018) 12:284–93. doi:10.1016/j.pmedr.2018.10.008
9. Nunes, BP, Flores, TR, Mielke, GI, Thume, E, and Facchini, LA. Multimorbidity and Mortality in Older Adults: a Systematic Review and Meta-Analysis. Arch Gerontol Geriatr (2016) 67:130–8. doi:10.1016/j.archger.2016.07.008
10. Makovski, TT, Schmitz, S, Zeegers, MP, Stranges, S, and van den Akker, M. Multimorbidity and Quality of Life: Systematic Literature Review and Meta-Analysis. Ageing Res Rev (2019) 53:100903. doi:10.1016/j.arr.2019.04.005
11. Foley, L, Larkin, J, Lombard-Vance, R, Murphy, AW, Hynes, L, Galvin, E, et al. Prevalence and Predictors of Medication Non-adherence Among People Living with Multimorbidity: a Systematic Review and Meta-Analysis. BMJ Open (2021) 11(9):e044987. doi:10.1136/bmjopen-2020-044987
12. Abebe, F, Schneider, M, Asrat, B, and Ambaw, F. Multimorbidity of Chronic Non-communicable Diseases in Low-And Middle-Income Countries: A Scoping Review. J Comorb (2020) 10:2235042X20961919. doi:10.1177/2235042X20961919
13. Pathirana, TI, and Jackson, CA. Socioeconomic Status and Multimorbidity: a Systematic Review and Meta‐analysis. Aust N Z J Public Health (2018) 42(2):186–94. doi:10.1111/1753-6405.12762
14. Seo, S, Kwon, YD, Yoo, K-B, Lee, Y, and Noh, J-W. Is Transient and Persistent Poverty Harmful to Multimorbidity?: Model Testing Algorithms. Int J Environ Res Public Health (2019) 16(13):2395. doi:10.3390/ijerph16132395
15. Hernández, B, Voll, S, Lewis, NA, McCrory, C, White, A, Stirland, L, et al. Comparisons of Disease Cluster Patterns, Prevalence and Health Factors in the USA, Canada, England and Ireland. BMC Public Health (2021) 21(1):1674–15. doi:10.1186/s12889-021-11706-8
16. Singer, L, Green, M, Rowe, F, Ben-Shlomo, Y, Kulu, H, and Morrissey, K. Trends in Multimorbidity, Complex Multimorbidity and Multiple Functional Limitations in the Ageing Population of England, 2002-2015.. J Comorb (2002–20152019) 9:2235042X19872030. doi:10.1177/2235042X19872030
17. Kunna, R, San Sebastian, M, and Stewart Williams, J. Measurement and Decomposition of Socioeconomic Inequality in Single and Multimorbidity in Older Adults in China and Ghana: Results from the WHO Study on Global AGEing and Adult Health (SAGE). Int J Equity Health (2017) 16(1):79–17. doi:10.1186/s12939-017-0578-y
18.The Academy of Medical Sciences. Multimorbidity: A Priority for Global Health Research (2018). https://acmedsci.ac.uk/file-download/82222577 (accessed May 24, 2022).
19.NIDS. What Is NIDS? (2020). http://www.nids.uct.ac.za/about/what-is-nids (accessed March 3, 2022).
20.NIDS. National Income Dynamics Study Wave 5 Overview 2008-2019 (2019). Available from: http://www.nids.uct.ac.za/images/documents/201901-NIDS-W5Overview-V1.0.pdf (Accessed March 3, 2022).
21. Cois, A, and Ehrlich, R. Antihypertensive Treatment and Blood Pressure Trends Among South African Adults: A Repeated Cross-Sectional Analysis of a Population Panel Survey. PLoS One (2018) 13(8):e0200606. doi:10.1371/journal.pone.0200606
22.Southern Africa Labour and Development Research Unit. National Income Dynamics Study 2017, Wave 5 [dataset]. Version 1.0.0. Pretoria: DataFirst (2018).
23. Brophy, T, Branson, N, Daniels, R, Leibbrandt, M, Mlatsheni, C, and Woolard, I. National Income Dynamics Study Panel User Manual. Version 1 (2018). Available from: http://www.nids.uct.ac.za/images/documents/20180831-NIDS-W5PanelUserManual-V1.0.pdf (Accessed May 18, 2022).
24.Southern Africa Labour and Development Research Unit. National Income Dynamics Study 2017, Wave 5 (2022). Available from: https://www.datafirst.uct.ac.za/dataportal/index.php/catalog/712 (accessed March 4, 2022).
25. Johnston, MC, Crilly, M, Black, C, Prescott, GJ, and Mercer, SW. Defining and Measuring Multimorbidity: a Systematic Review of Systematic Reviews. Eur J Public Health (2018) 29(1):182–9. doi:10.1093/eurpub/cky098
26. Holzer, BM, Siebenhuener, K, Bopp, M, and Minder, CE. Evidence-based Design Recommendations for Prevalence Studies on Multimorbidity: Improving Comparability of Estimates. Popul Health Metr (2017) 15(1):9. doi:10.1186/s12963-017-0126-4
27. Chobanian, AV, Bakris, GL, Black, HR, Cushman, WC, Green, LA, Izzo, JL, et al. The Seventh Report of the Joint National Committee on Prevention, Detection, Evaluation, and Treatment of High Blood Pressure: the JNC 7 Report. JAMA (2003) 289(19):2560–72. doi:10.1001/jama.289.19.2560
28. Xu, X, Mishra, GD, and Jones, M. Evidence on Multimorbidity from Definition to Intervention: An Overview of Systematic Reviews. Ageing Res Rev (2017) 37:53–68. doi:10.1016/j.arr.2017.05.003
29.National Department of Health. Statistics South Africa, South African Medical Research Council, and ICF. South Africa Demographic and Health Survey 2016. Pretoria, South Africa, and Rockville, Maryland, USA: NDoH, Stats SA, SAMRC, and ICF (2019). Available from: https://dhsprogram.com/pubs/pdf/FR337/FR337.pdf (accessed May 24, 2022).
30.The DHS Program. Wealth index (2022). Available from: https://dhsprogram.com/topics/wealth-index/(accessed April 5, 2022).
31. Bradshaw, D, Joubert, J, and Abdelatif, N. Estimating the Changing Disease burden Attributable to High Body Mass index for South Africa for 2000, 2006 and 2012. Afr Med J (2022). In Press.
32. Branson, N, and Wittenberg, M. Longitudinal and Cross-Sectional Weights in the NIDS Data 1–5 [NIDS Technical Paper Number 9]. In: Southern Africa Labour and Development Research Unit. Cape Town, South Africa: University of Cape Town (2019). p. 3.
33.UN Department of International Economic and Social Affairs. Provisional Guidelines on Standard International Age Classifications. New York: UN (1982). Available from: http://digitallibrary.un.org/record/32113/files/ST_ESA_STAT_SER.M_74-EN.pdf (Accessed March 4, 2022).
34.StataCorp. Stata User's Guide: Release 17 (2021). Available from: https://www.stata.com/manuals/u.pdf July 23, 2021).Accessed
35. Pregibon, D. Logistic Regression Diagnostics. Ann Statist (1981) 9(4):705–24. doi:10.1214/aos/1176345513
36. Weller, BE, Bowen, NK, and Faubert, SJ. Latent Class Analysis: A Guide to Best Practice. J Black Psychol (2020) 46(4):287–311. doi:10.1177/0095798420930932
37.The Methodology Center. LCA Stata Plugin. Version 1.2. Penn State: University Park: The Methodology Center (2015).
38. Lanza, ST, Dziak, JJ, Huang, L, Wagner, AT, and Collins, LM. LCA Stata Plugin Users’ Guide. Version 1.2 (2015). Available from: https://www.methodology.psu.edu/files/2019/03/Stata-LCA-Plugin-v1.2c-2e00dl9.pdf (accessed May 24, 2022).
39. Schwarz, G. Estimating the Dimension of a Model. Ann Statist (1978) 6:461–4. doi:10.1214/aos/1176344136
40. Sclove, SL. Application of Model-Selection Criteria to Some Problems in Multivariate Analysis. Psychometrika (1987) 52(3):333–43. doi:10.1007/bf02294360
41. Akaike, H. Information Theory as an Extension of the Maximum Likelihood Principle. In: BN Petrov, and F Csaki, editors. Selected Papers of Hirotugu Akaike. Springer Series in Statistics. New York, NY: Springer (1998).
42. Nylund, KL, Asparouhov, T, and Muthén, BO. Deciding on the Number of Classes in Latent Class Analysis and Growth Mixture Modeling: A Monte Carlo Simulation Study. Struct Equation Model A Multidisciplinary J (2007) 14(4):535–69. doi:10.1080/10705510701575396
43. Asogwa, OA, Boateng, D, Marzà-Florensa, A, Peters, S, Levitt, N, van Olmen, J, et al. Multimorbidity of Non-communicable Diseases in Low-Income and Middle-Income Countries: a Systematic Review and Meta-Analysis. BMJ Open (2022) 12(1):e049133. doi:10.1136/bmjopen-2021-049133
44. Stats, SA. Protecting South Africa's Elderly (2020). Available from: https://www.statssa.gov.za/?p=13445 (accessed April 21, 2022).
45. Daviaud, E, Kelly, G, Cornell, J, Geffen, L, and Solanki, G. Population Ageing in South Africa: Trends, Impact, and Challenges for the Health Sector. S Afr Health Rev. 2019;2019(1):175–82. doi:10.10520/EJC-1d2b0b4f5a
46.World Health Organization Regional Office for Europe. Health through Life Stages (2022). Available from: https://www.euro.who.int/en/health-topics/Life-stages/pages/life-course (accessed May 10, 2022).
47. Odland, ML, Payne, C, Witham, MD, Siedner, MJ, Barnighausen, T, Bountogo, M, et al. Epidemiology of Multimorbidity in Conditions of Extreme Poverty: a Population-Based Study of Older Adults in Rural Burkina Faso. BMJ Glob Health (2020) 5(3):e002096. doi:10.1136/bmjgh-2019-002096
48. Alaba, O, and Chola, L. The Social Determinants of Multimorbidity in South Africa. Int J Equity Health (2013) 12(1):63. doi:10.1186/1475-9276-12-63
49. Goetjes, E, Pavlova, M, Hongoro, C, and Groot, W. Socioeconomic Inequalities and Obesity in South Africa-A Decomposition Analysis. Int J Environ Res Public Health (2021) 18(17):9181. doi:10.3390/ijerph18179181
50. Alaba, O, and Chola, L. Socioeconomic Inequalities in Adult Obesity Prevalence in South Africa: a Decomposition Analysis. Int J Environ Res Public Health (2014) 11(3):3387–406. doi:10.3390/ijerph110303387
51. Adeboye, B, Bermano, G, and Rolland, C. Obesity and its Health Impact in Africa: a Systematic Review. Cardiovasc J Afr (2012) 23(9):512–21. doi:10.5830/cvja-2012-040
52. Ataguba, J. Health Care Financing in South Africa: Moving towards Universal Coverage. Continuing Med Education (2010) 28(2).
53. Roomaney, RA, van Wyk, B, Turawa, EB, and Pillay-van Wyk, V. Multimorbidity in South Africa: a Systematic Review of Prevalence Studies. BMJ Open (2021) 11(10):e048676. doi:10.1136/bmjopen-2021-048676
54. Chidumwa, G, Maposa, I, Corso, B, Minicuci, N, Kowal, P, Micklesfield, LK, et al. Identifying Co-occurrence and Clustering of Chronic Diseases Using Latent Class Analysis: Cross-Sectional Findings from SAGE South Africa Wave 2. BMJ Open (2021) 11(1):e041604. doi:10.1136/bmjopen-2020-041604
55. Mohan, V, Seedat, YK, and Pradeepa, R. The Rising burden of Diabetes and Hypertension in Southeast Asian and African Regions: Need for Effective Strategies for Prevention and Control in Primary Health Care Settings. Int J Hypertens (2013) 2013:409083. doi:10.1155/2013/409083
56. Tesfaye, B, Alebel, A, Gebrie, A, Zegeye, A, Tesema Leshargie, C, Ferede, A, et al. Diabetes Mellitus and its Association with Hypertension in ethiopia: a Systematic Review and Meta-Analysis. Diabetes Res Clin Pract (2019) 156:107838. doi:10.1016/j.diabres.2019.107838
57. Prados-Torres, A, Calderón-Larrañaga, A, Hancco-Saavedra, J, Poblador-Plou, B, and van den Akker, M. Multimorbidity Patterns: a Systematic Review. J Clin Epidemiol (2014) 67(3):254–66. doi:10.1016/j.jclinepi.2013.09.021
58. Ekoru, K, Doumatey, A, Bentley, AR, Chen, G, Zhou, J, Shriner, D, et al. Type 2 Diabetes Complications and Comorbidity in Sub-saharan Africans. EClinicalMedicine (2019) 16:30–41. doi:10.1016/j.eclinm.2019.09.001
59. Roomaney, RA, van Wyk, B, Cois, A, and Pillay-van Wyk, V. Multimorbidity Patterns in a National HIV Survey of South African Youth and Adults. Front Public Health (2022) 10:862993. doi:10.3389/fpubh.2022.862993
60.World Health Organization. Hypertension (2022). Available from: https://www.who.int/health-topics/hypertension#tab=tab_1 (accessed April 21, 2022).
61. Fuchs, FD, and Whelton, PK. High Blood Pressure and Cardiovascular Disease. Hypertension (2020) 75(2):285–92. doi:10.1161/HYPERTENSIONAHA.119.14240
62. Zhou, B, Carrillo-Larco, RM, and Danaei, G. Worldwide Trends in Hypertension Prevalence and Progress in Treatment and Control from 1990 to 2019: a Pooled Analysis of 1201 Population-Representative Studies with 104 Million Participants. Lancet (2021) 398(10304):957–80. doi:10.1016/S0140-6736(21)01330-1
63. Rijken, M, Hujala, A, van Ginneken, E, Melchiorre, MG, Groenewegen, P, and Schellevis, F. Managing Multimorbidity: Profiles of Integrated Care Approaches Targeting People with Multiple Chronic Conditions in Europe. Health Policy (2018) 122(1):44–52. doi:10.1016/j.healthpol.2017.10.002
64.World Health Organization. Integrated Care for Older People: Realigning Primary Health Care to Respond to Population Ageing. Geneva, Switzerland: World Health Organization (2018).
65. Afshar, S, Roderick, PJ, Kowal, P, Dimitrov, BD, and Hill, AG. Multimorbidity and the Inequalities of Global Ageing: a Cross-Sectional Study of 28 Countries Using the World Health Surveys. BMC Public Health (2015) 15(1):776. doi:10.1186/s12889-015-2008-7
66. Sharman, M, and Bachmann, M. Prevalence and Health Effects of Communicable and Non-communicable Disease Comorbidity in Rural KwaZulu-Natal, South Africa. Trop Med Int Health (2019) 24(10):1198–207. doi:10.1111/tmi.13297
67. Weimann, A, Dai, D, and Oni, T. A Cross-Sectional and Spatial Analysis of the Prevalence of Multimorbidity and its Association with Socioeconomic Disadvantage in South Africa: A Comparison between 2008 and 2012. Soc Sci Med (2016) 163:144–56. doi:10.1016/j.socscimed.2016.06.055
68. Nojilana, B, Peer, N, and Abdelatif, N. Estimating the Changing burden of Disease Attributable to High Blood Pressure in South Africa for 2000, 2006 and 2012. S Afr Med J (2022). In Press.
69. Shisana, O, Labadarios, D, Rehle, T, Simbayi, L, Zuma, KK, Reddy, SP, et al. The South African National Health and Nutrition Examination Survey, 2012: SANHANES-1: The Health and Nutritional Status of the Nation. South Africa: HSRC press (2014).
70. Pillay van Wyk, V, Cois, A, and Kegne, A. Estimating the Changing Disease burden Attributable to High Fasting Plasma Glucose in South Africa for 2000, 2006 and 2012. S Afr Med J (2022). In Press.
Keywords: multimorbidity, disease patterns, latent class analysis, wealth index, South Africa
Citation: Roomaney RA, van Wyk B, Cois A and Pillay-van Wyk V (2022) Inequity in the Distribution of Non-Communicable Disease Multimorbidity in Adults in South Africa: An Analysis of Prevalence and Patterns. Int J Public Health 67:1605072. doi: 10.3389/ijph.2022.1605072
Received: 20 May 2022; Accepted: 18 July 2022;
Published: 16 August 2022.
Edited by:
Salvatore Panico, University of Naples Federico II, ItalyCopyright © 2022 Roomaney, van Wyk, Cois and Pillay-van Wyk. This is an open-access article distributed under the terms of the Creative Commons Attribution License (CC BY). The use, distribution or reproduction in other forums is permitted, provided the original author(s) and the copyright owner(s) are credited and that the original publication in this journal is cited, in accordance with accepted academic practice. No use, distribution or reproduction is permitted which does not comply with these terms.
*Correspondence: R. A. Roomaney, cmlmcWFoLnJvb21hbmV5QG1yYy5hYy56YQ==